❓ Frequently Asked Questions
What is the point estimate and confidence interval?
A point estimate is an average estimate of a quantity of interest, such as revenue attributable to a particular channel.
A confidence interval is a range we expect the true quantity to lie in. The range helps us understand the precision of our estimate.
How does the optimizer allocate spend across time in a particular channel?
The optimizer looks at past performance trends when optimizing future spend. It incorporates two different measurements:
Seasonal trends in baseline performance in the channel - e.g. is the channel generally better in Q4 compared to other quarters?
Global saturation changes, both seasonal and from saturation spikes - e.g. a saturation spike present in Q4 would mean that the channel saturates more slowly and that marketing in the channel remains effective at higher than usual spend levels.
The optimizer assumes that each day is similar to the next as well as that each day is similar to the same day the previous year. The optimizer applies these assumptions and makes predictions about when your marketing spend into each channel will be the most effective to determine your spend flighting recommendations.
In the absence of seasonal changes in channel saturation or baseline performance, the optimizer will tend to spread spend evenly in order to reduce channel saturation at any given point.
How is uncertainty accounted for in the optimizer?
When managing your marketing channels, it's similar to managing an investment portfolio. Each channel has different levels of risk and return, and we want to maximize the chances of hitting your overall goals by carefully balancing these factors.
Some channels tend to be highly correlated with each other, meaning they move similarly, and this can increase risk. The optimizer takes this into account by reducing spend in "high-risk" channels to prevent over-reliance on them.
Essentially, we want to spread out the risk, which is reflected in how wide your uncertainty interval is. The optimizer considers uncertainty as a multiplier and gives less weight to riskier channels. This ensures we’re not putting too much budget into areas with high uncertainty.
The goal is to create an optimal portfolio that balances risk with the potential return on your key performance indicators (KPIs).
How to interpret correlation between channels?
Correlation between channels is when the model is unable to parse out the KPI driven between two or more channels.
When there are two channels that have very similar historical spend patterns, the model often can not tell how much of the KPI was driven by each channel. This leads to the channels being correlated.
Each correlated channel then might have a high uncertainty but could have a low uncertainty together (ChannelA+Channel B).
How can we account for any promotions, and seasonality so that the results are not confounded when running tests?
Spikes caused by promotions or seasonality can sometimes confound the results of a spend experiment as an increase in spend might coincide with a period of decreased saturation. This could mean that the signal gained from the test may not be applicable to your business during ‘normal times’.
When planning a go-dark or spend-up test, run the following checks to make sure your results are actionable:
Check the spikes graph in the insights page to make sure that there are no historical spikes you expect again during the test period
Check with your promotions team to make sure they are not planning any promotions for the time period of the experiment
Check the channel summary graphs to see if there are any trends in the historical ROI that coincide with with your test period. Does the ROI increase every summer? Maybe you should wait until the fall to plan a spend test.
When planning a GeoLift test, the main consideration to keep in mind is that GeoLift assumes that the only factor changing across geographies is the spend. Anything that violates this assumption has the potential to confound your results.
Make sure there are no geography specific promotions or spikes during the test period.
How does adding new data impact the revision for last years ROI?
Every time data is added to the model, the model is retrained with all the historical data. Recast keeps 2 years worth of data in the model so when a week of data is added, the first week of data in the model is dropped and the model is re-estimated. This is to make sure the model stays trained on up to date data.
This could result in revisions in the model’s past estimates. As we update our estimates of seasonality and time shifts from the new data, that will have a retroactive influence on the old data. New data could reveal new seasonal patterns. These are applied retroactively to prior years to better explain historical data.
At Recast we have multiple checks that we run to ensure a high stability score. This should reassure you that the model is learning and improving its estimates with new data.
Why is there higher uncertainty when we increased spend?
There are several reasons why you could be seeing higher uncertainty when scaling up spend.
Firstly, this could be because you have been spending pretty consistently in each of your channels historically. This means the model does not have much information about your channel saturation at higher spend levels. This can lead to increased uncertainty when you increase your spend as the model is still in the process of learning about your channel’s saturation.
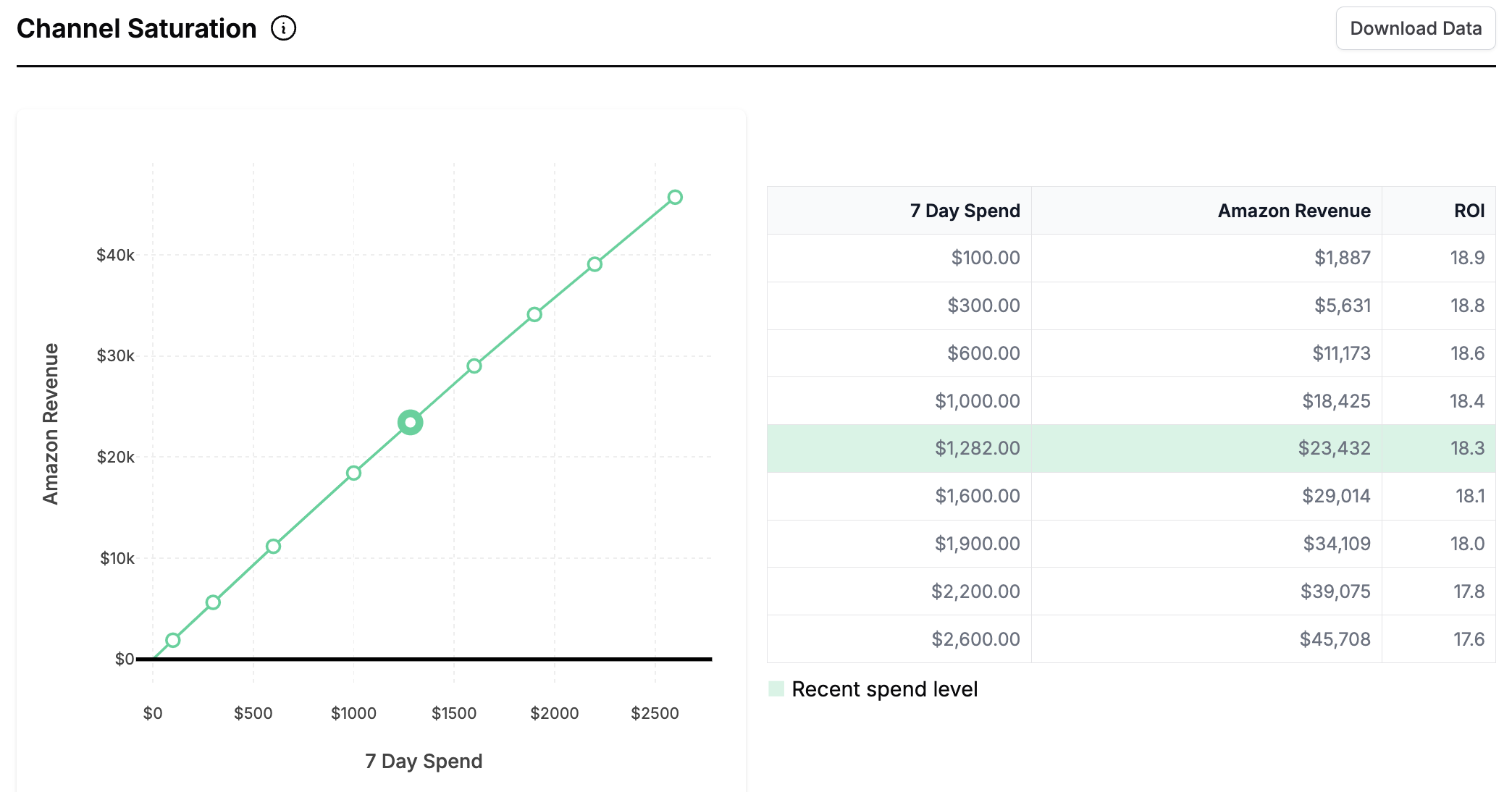
Additionally, channels with a longer time shift might initially display higher uncertainty when spend is increased as the model does not see the effects of the increase until much later.
Moreover, if you find that you are consistently scaling up all your channels during peak time, it may be that the model is unable to differentiate the effects of spend in the various channels.
You can use the variance decomposition report to find out where your uncertainty is coming from. If the uncertainty is coming from correlation with other channels, we can help you plan a go dark test or a spend up test to help the model differentiate between your various channels.