π§π½βπ« Insights
The first place many people start is on the Insights tab. The Insights tab tells us about whatβs been happening in the past and shows the results of a statistical model trained on your companyβs historical data.
Use the insights pages to understand how your marketing has been performing recently. Identify trends using the overview page, check in on your backtests and dive deep into your channels' performance.
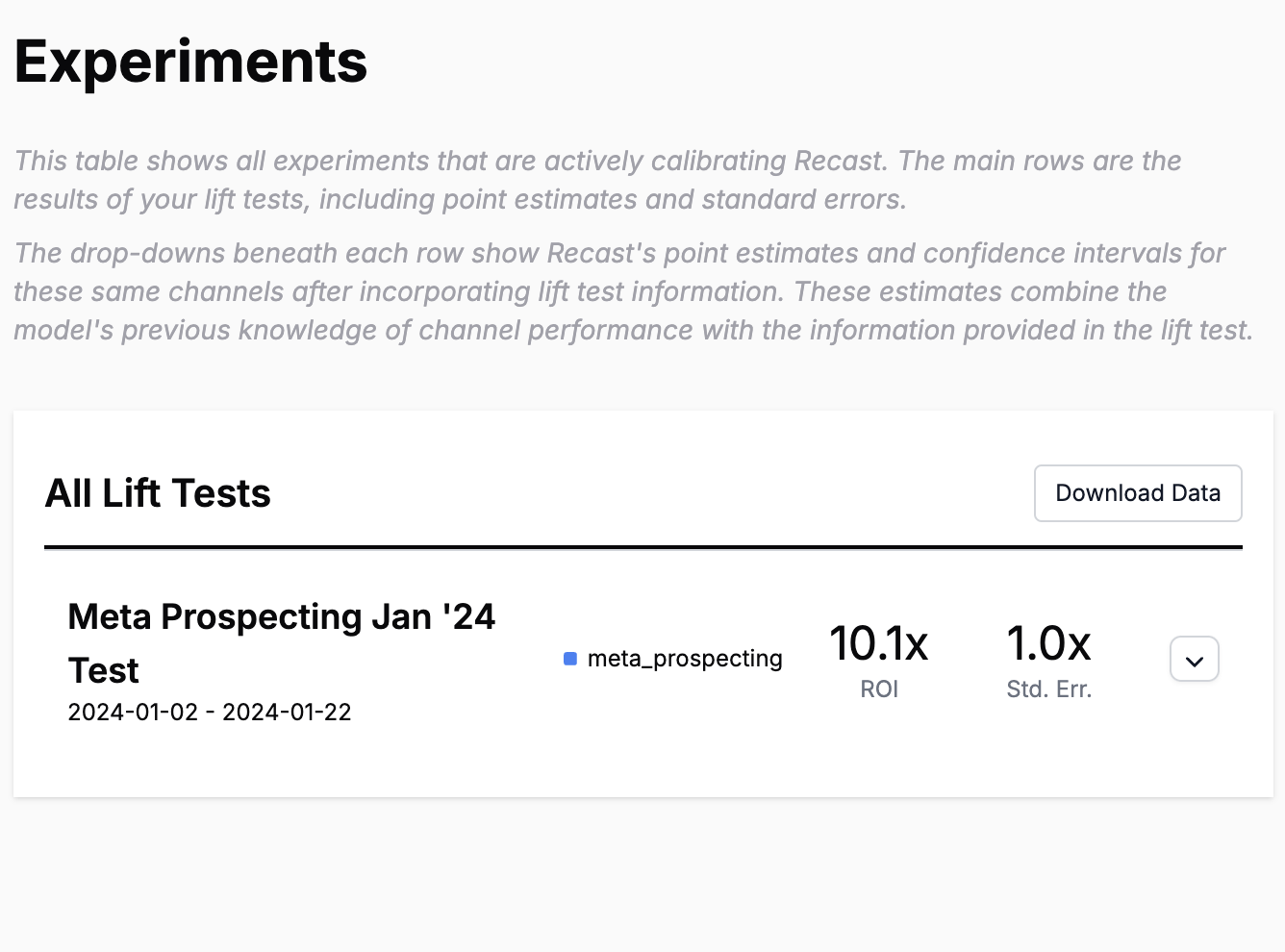
View all your the lift tests included in your model.
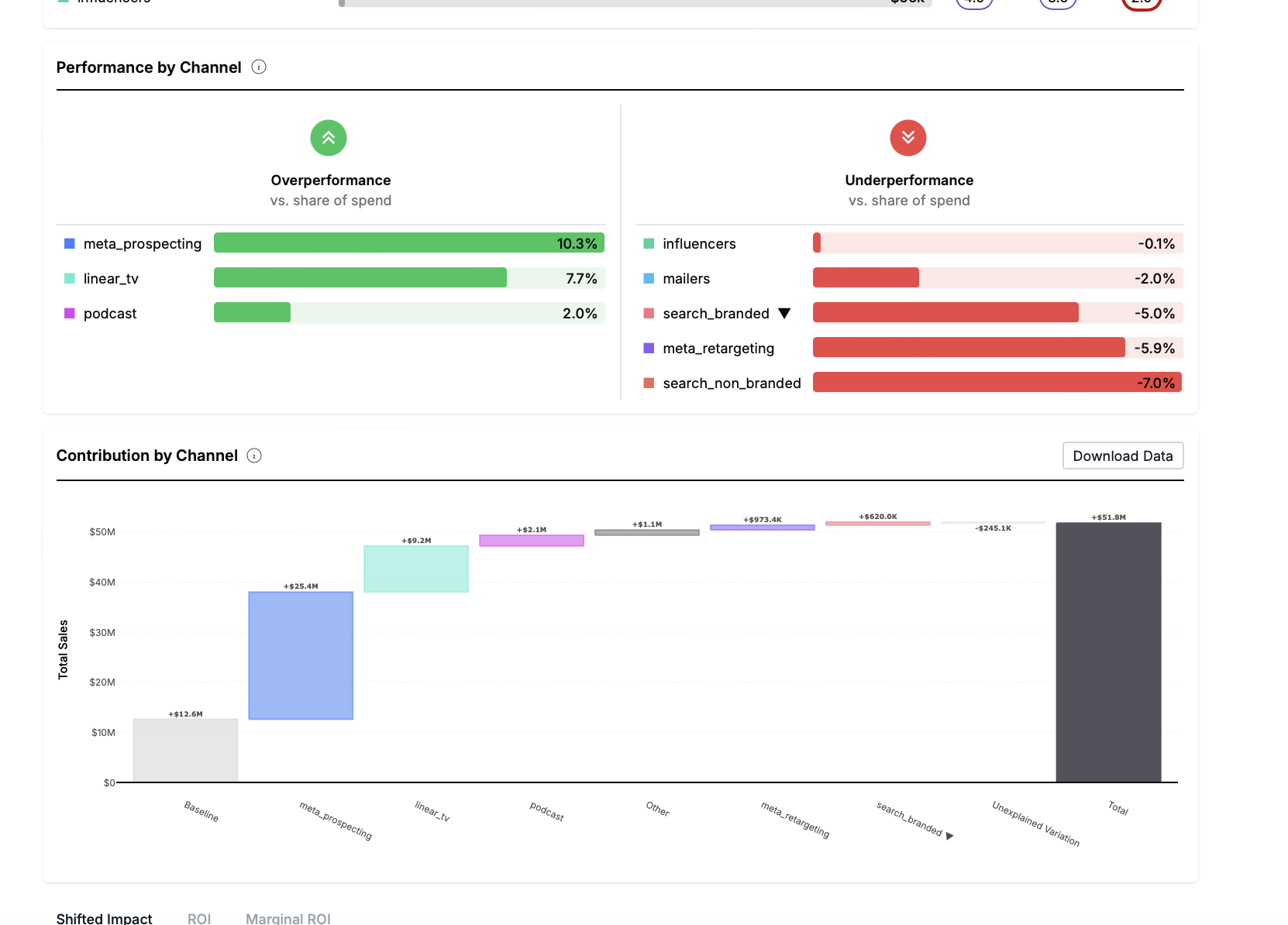
Get a snapshot of your overall marketing performance.
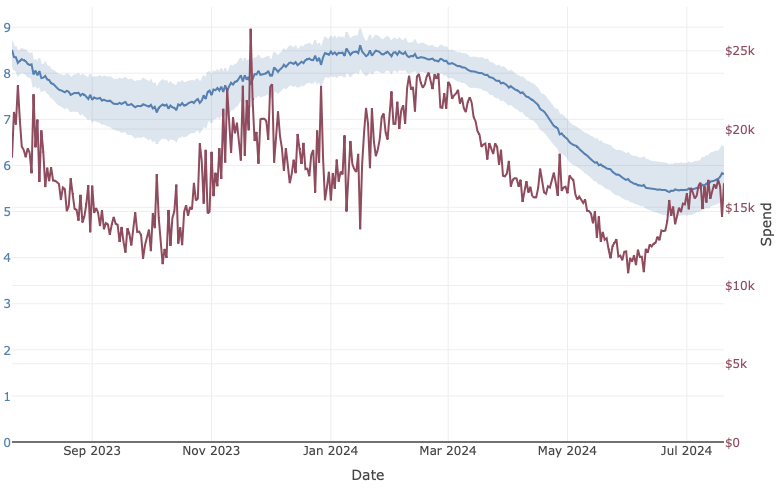
Analyze the performance of each of your channels.
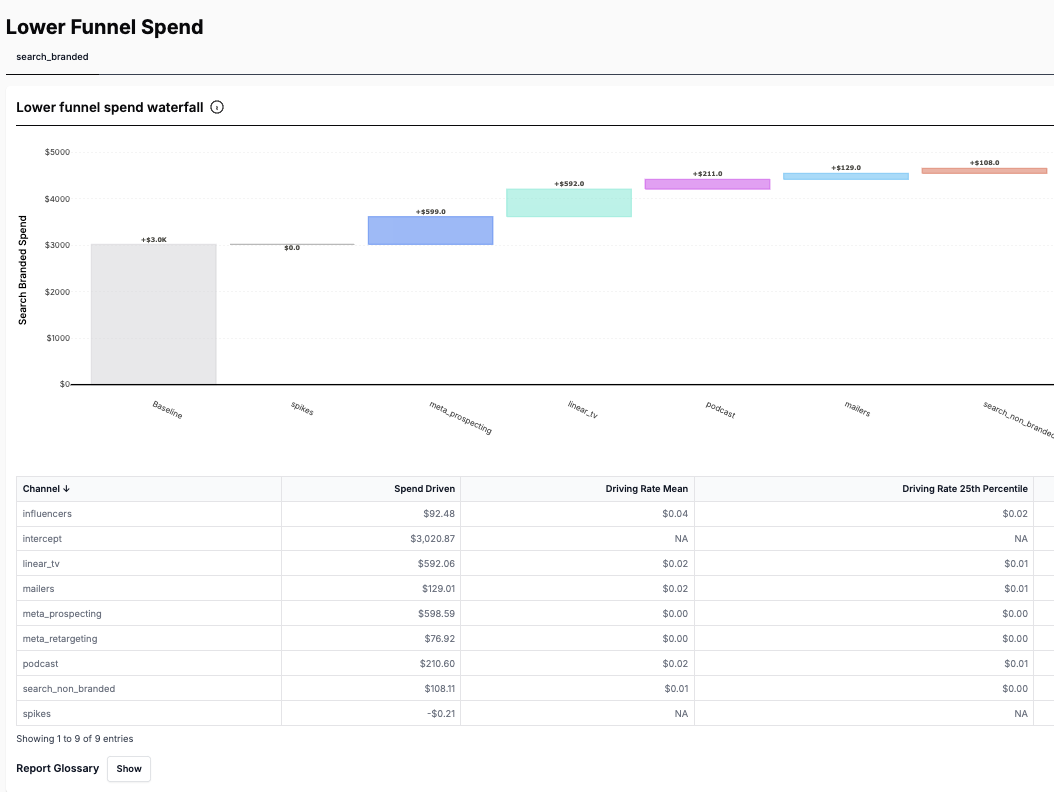
Measure the performance of your lower funnel channels.
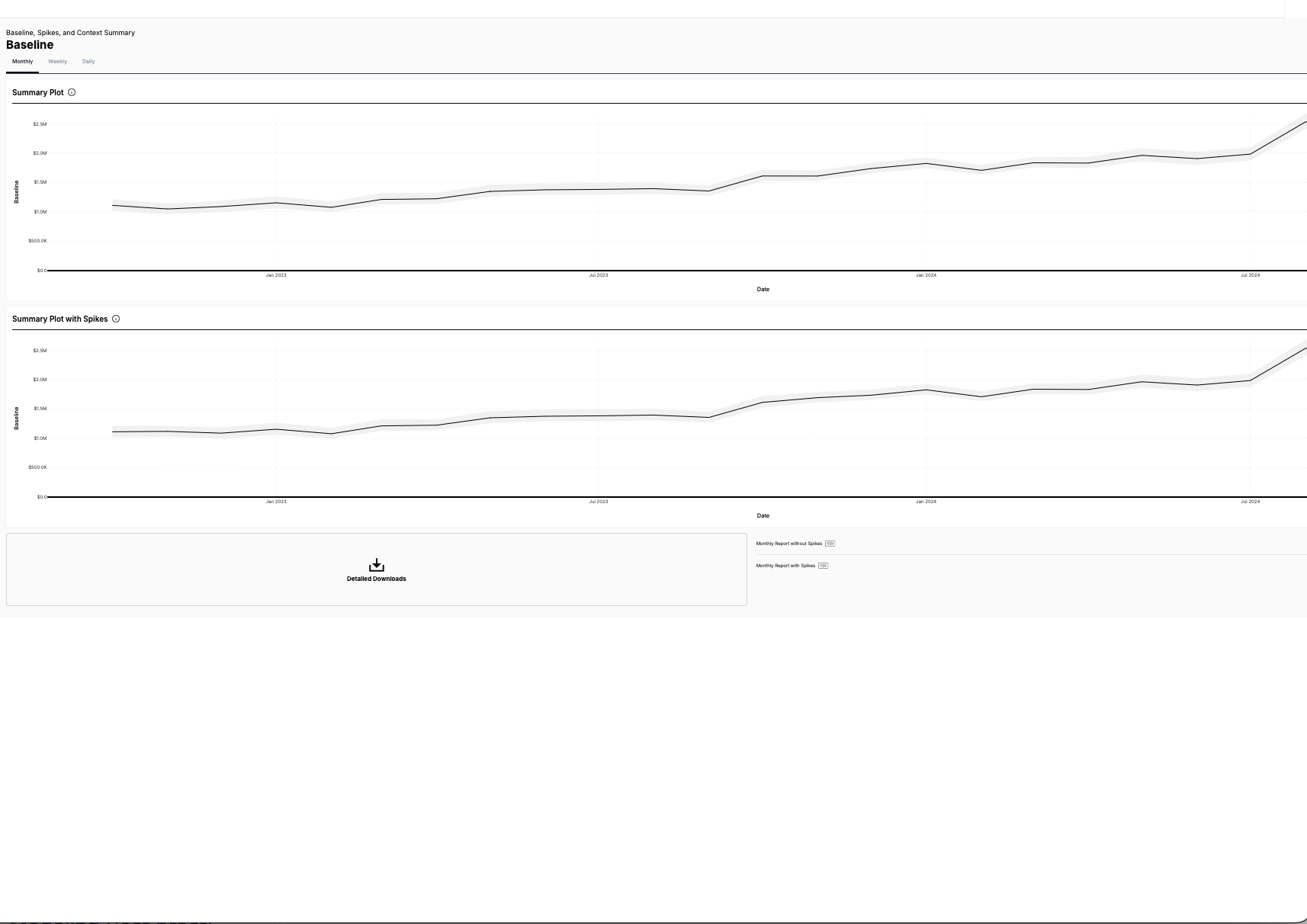
Measure your organic performance.
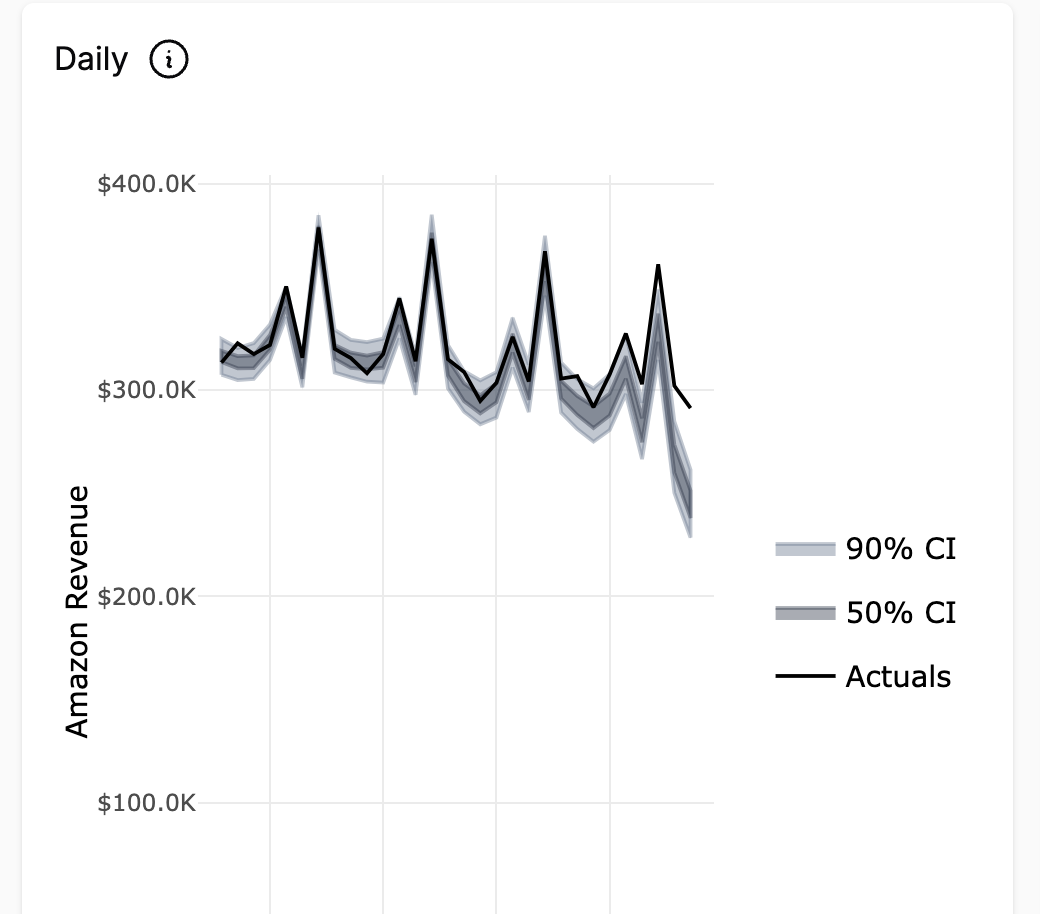
Review the forecasting accuracy of your model.